Image Recovery and Recognition via Exploiting the Structural Bias of Neural Networks
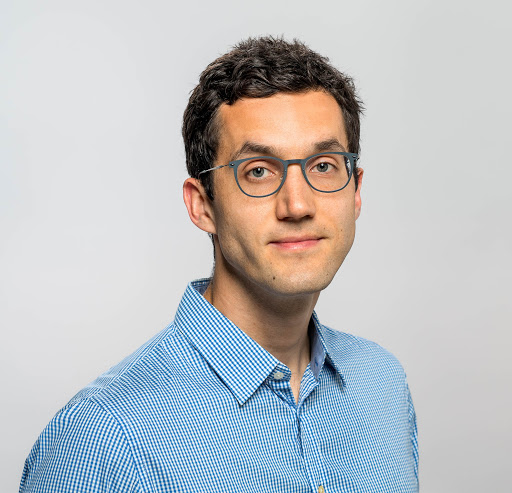
Guest Speaker:
Reinhard Heckel — Technical University of Munich, Germany
Wednesday, February 19, 2020
EEB 132
2:00PM
ABSTRACT: Deep neural networks are highly successful tools for image classification, recovery, and restoration. This success is often attributed to large amounts of training data. However, recent findings challenge this view and instead suggest that a major contributing factor to this success is that the architecture imposes strong prior assumptions-”so strong that it enables image recovery without any training data. In this talk we discuss two instances of this phenomena: First, we show that fitting a convolutional network to a corrupted and/or under-sampled measurement of an image provably removes noise and corruptions from that image, without ever having trained the network. Second, we show that it is possible to learn from a dataset with both true and false examples, obtained without explicit human annotations, by exploiting the phenomena that neural networks fit true examples faster than false ones.
BIO: Reinhard Heckle is the Rudolf Moessbauer assistant professor in the Department of Electrical and Computer Engineering (ECE) at the Technical University of Munich, and an adjunct assistant professor in the ECE department at Rice University, where he was an assistant professor before, from 2017-2019. Before that, he spent one and a half years as a postdoctoral researcher in the Department of Electrical Engineering and Computer Sciences at the University of California, Berkeley, and a year in the Cognitive Computing & Computational Sciences Department at IBM Research, Zurich. He completed my PhD in electrical engineering in 2014 at ETH Zurich and was a visiting PhD student at the Statistics Department at Stanford University. He is working at the intersection of machine learning and signal/information processing with a current focus on deep networks for solving inverse problems, learning from few and noisy samples, and DNA data storage.
Hosted by: Mahdi Soltanolkotabi