Circuit-Based Intrinsic Methods to Detect Overfitting
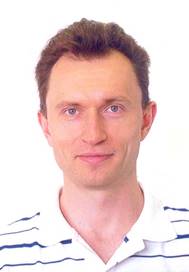
Guest Speaker:
Alan Mishchenko – Dept. of Electrical Engineering and Computer Sciences, University of California, Berkeley
Wednesday, September 9th, 2020
Zoom Webinar Link
2:00pm – 3:00pm
Webinar Link:
https://usc.zoom.us/webinar/register/WN_YSl0DRVOQJetWGNAACPOYQ
Abstract: The focus of this talk is on intrinsic methods to detect overfitting. By intrinsic methods, we mean methods that rely only on the model and the training data, as opposed to traditional methods that rely on performance on a test set or on bounds from model complexity. We propose a family of intrinsic methods, called Counterfactual Simulation (CFS), which analyze the flow of training examples through the model by identifying and perturbing rare patterns. By applying CFS to logic circuits we get a method that has no hyper-parameters and works uniformly across different types of models such as neural networks, random forests and lookup tables. Experimentally, CFS can separate models with different levels of overfit using only their logic circuit representations without any access to the high level structure. By comparing lookup tables, neural networks, and random forests using CFS, we get insight into why neural networks generalize. The paper appeared at ICML 2020: https://people.eecs.berkeley.edu/~alanmi/publications/2020/icml20_cfs.pdf
Biography: Alan graduated with M.S. from Moscow Institute of Physics and Technology (Moscow, Russia) in 1993 and received his Ph.D. from Glushkov Institute of Cybernetics (Kiev, Ukraine) in 1997. In 2002, Alan joined the EECS Department at the University of California, Berkeley, where he is currently a full researcher. His research is in computationally efficient logic synthesis and formal verification.
—
Host: Pierluigi Nuzzo
Center for Cyber-Physical Systems and the Internet of Things (CCI) http://cci.usc.edu
Ming Hsieh Institute for Electrical and Computer Engineering (MHI) http://mhi.usc.edu
—